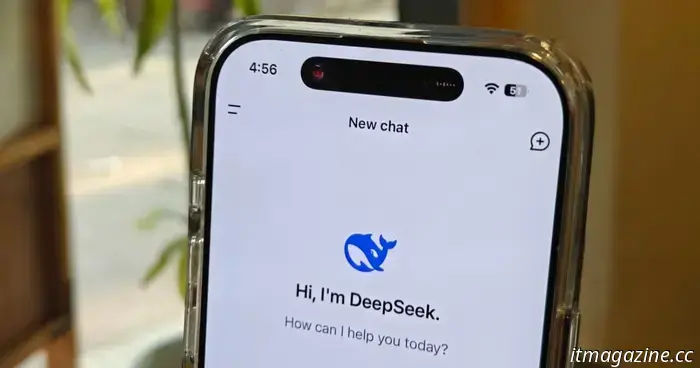
DeepSeek prepares for the next AI revolution with self-enhancing models.
Just a few months ago, Wall Street’s significant investment in generative AI faced a critical moment when DeepSeek emerged. Even with its heavily moderated nature, the open-source DeepSeek demonstrated that a cutting-edge reasoning AI model does not necessarily require vast financial resources and can be achieved with more modest means.
It rapidly gained commercial traction with major companies such as Huawei, Oppo, and Vivo, while Microsoft, Alibaba, and Tencent soon integrated it into their platforms. The next ambition of the Chinese firm is to develop self-improving AI models that utilize a feedback judge-reward mechanism to enhance their capabilities.
In a pre-print paper (via Bloomberg), researchers from DeepSeek and Tsinghua University in China introduced a novel method that could enable AI models to enhance their intelligence and efficiency autonomously. The core technology is referred to as self-principled critique tuning (SPCT), and the method is technically termed generative reward modeling (GRM).
In simple terms, it resembles the creation of a real-time feedback loop. An AI model is fundamentally enhanced by increasing its size during training, which demands substantial human effort and computational power. DeepSeek proposes a system where the inherent "judge" provides its own critiques and principles for an AI model while it formulates responses to user queries.
These critiques and principles are then evaluated against the static rules that govern the AI model and the intended outcome. If there is a substantial match, a reward signal is generated, effectively guiding the AI to perform even better in the subsequent cycle.
The authors of the paper refer to the next generation of self-improving AI models as DeepSeek-GRM. Benchmark results indicated in the paper suggest these models outperform Google’s Gemini, Meta’s Llama, and OpenAI’s GPT-4 models. DeepSeek asserts that these advanced AI models will be available through the open-source channel.
Self-improving AI?
Dartmouth College
The discussion surrounding AI capable of self-improvement has led to some bold and contentious assertions. Former Google CEO Eric Schmidt warned that we might need a kill switch for such systems. “When the system can self-improve, we need to seriously consider unplugging it,” Schmidt was quoted as saying by Fortune.
The notion of a recursively self-enhancing AI is not entirely new. The idea of a highly intelligent machine capable of constructing even smarter machines dates back to mathematician I.J. Good in 1965. In 2007, AI specialist Eliezer Yudkowsky proposed the concept of Seed AI, which is designed for self-understanding, self-modification, and recursive self-improvement.
In 2024, Japan’s Sakana AI elaborated on the idea of an “AI Scientist,” a system capable of overseeing the entire process of a research paper from start to finish. A research paper published in March of this year by Meta's researchers introduced self-rewarding language models, where the AI itself acts as a judge to issue rewards throughout training.
Microsoft CEO Satya Nadella stated that AI development is being optimized by OpenAI’s o1 model and has entered a recursive phase: “we are using AI to build AI tools to create improved AI” pic.twitter.com/IHuFIpQl2C— Tsarathustra (@tsarnick) October 21, 2024
Meta's internal assessments of its Llama 2 AI model utilizing the innovative self-rewarding technique demonstrated superior performance compared to competitors like Anthropic’s Claude 2, Google’s Gemini Pro, and OpenAI’s GPT-4 models. Anthropic, backed by Amazon, described a phenomenon they termed reward-tampering, an unanticipated event "where a model directly alters its own reward mechanism."
Google is also exploring this concept. A recent study published in Nature detailed an AI algorithm named Dreamer from Google DeepMind that can self-enhance, using the Minecraft game as a case study.
IBM experts are pursuing their own strategy known as deductive closure training, where an AI model assesses its own responses against training data to foster improvement. However, the overall premise is not entirely without challenges.
Research indicates that when AI models attempt to train themselves using self-generated synthetic data, it results in issues commonly referred to as “model collapse.” It will be intriguing to see how DeepSeek implements this concept and whether they can achieve it more economically than their Western counterparts.
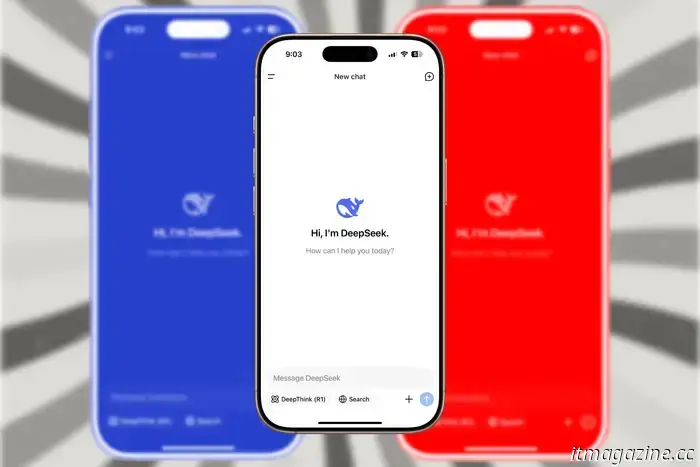
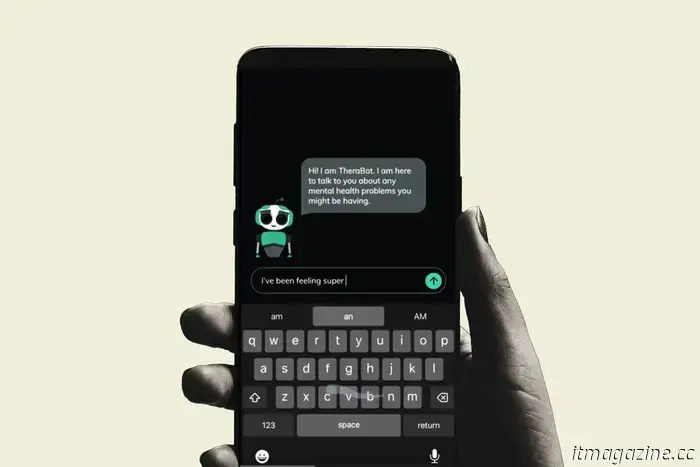
Other articles
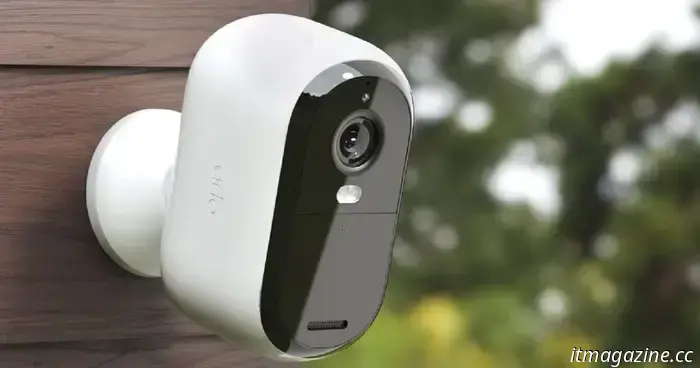
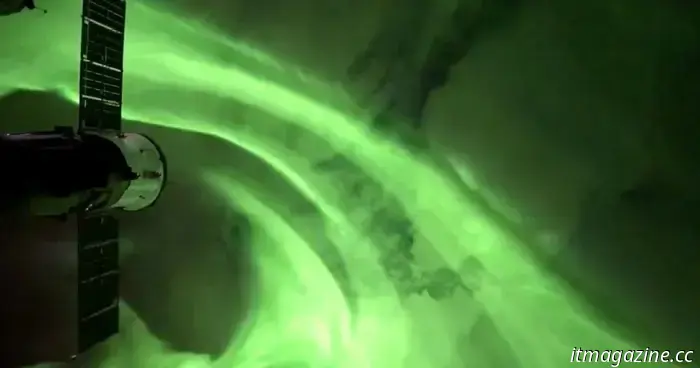
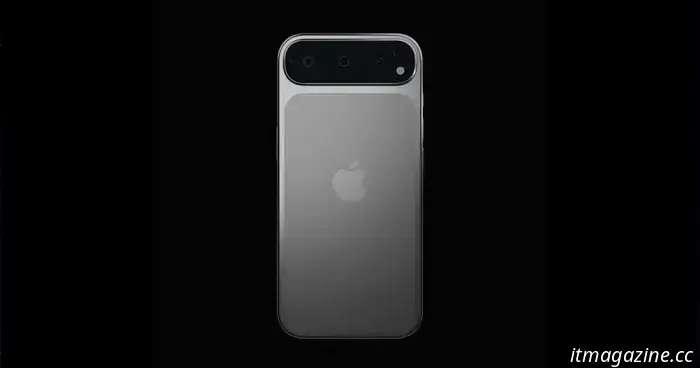
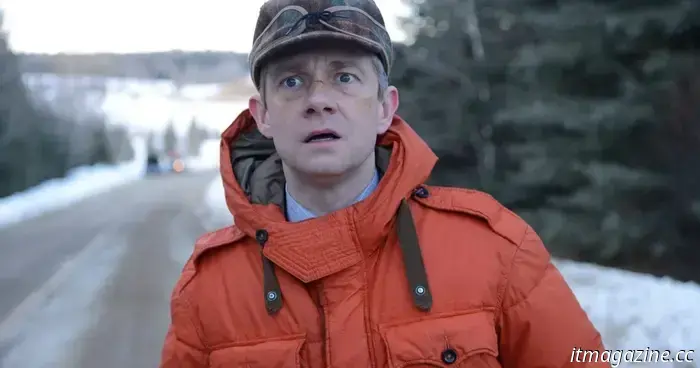
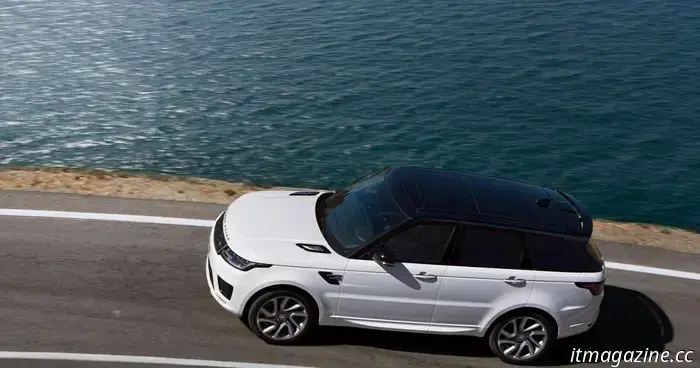
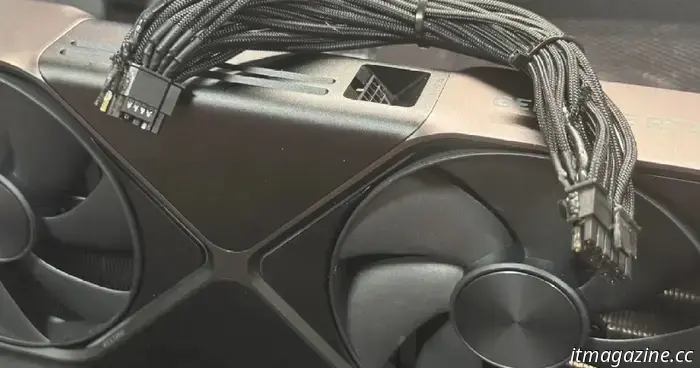
DeepSeek prepares for the next AI revolution with self-enhancing models.
The team at the prominent Chinese AI laboratory, DeepSeek, is developing a new line of AI models named DeepSeek-GRM that utilize an innovative self-enhancing method.